What is Augmented Analytics? Definition, Examples, and Tools
- -
- Time -
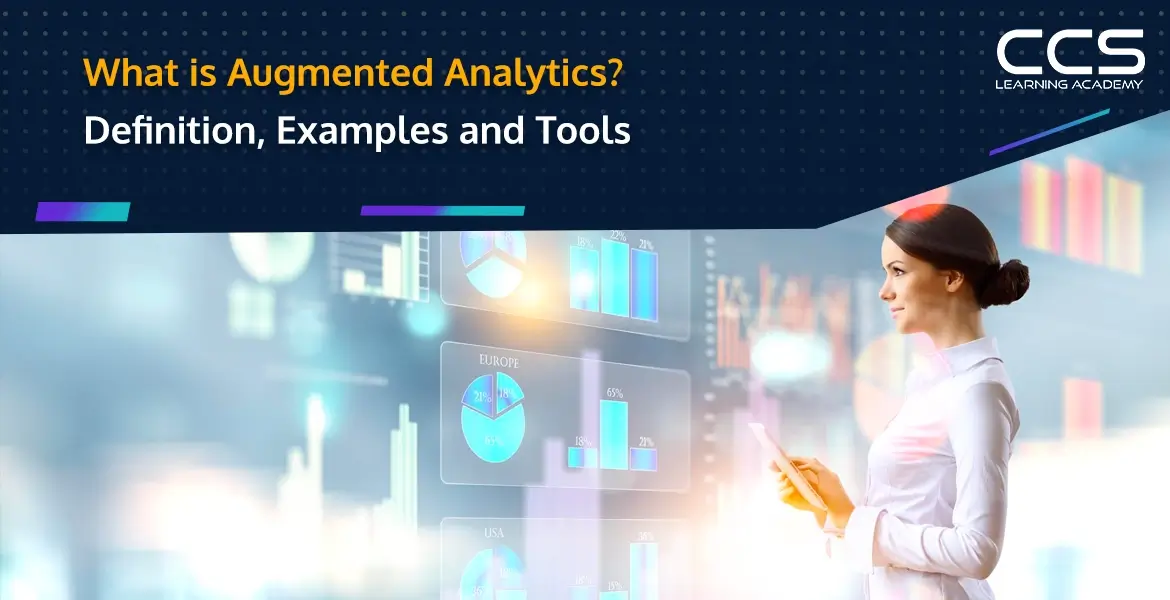
Augmented analytics integrates machine learning into BI tools for faster data preparation, analysis, and reporting – enabling deeper insights for users of all skill levels.
The volume and complexity of data that organizations need to analyze continue to rise exponentially. Making timely decisions based on accurate intelligence requires democratizing access to such insights across the enterprise. This is where augmented analytics comes in – integrating artificial intelligence and machine learning seamlessly into business intelligence platforms.
Let’s explore what augmented analytics entails, real-world applications, and top tools in this space, as well as how it helps unlock value through intelligent analytics automation.
What is Augmented Analytics?
Augmented analytics refers to business intelligence and data visualization software that utilizes machine learning and natural language processing to assist users in data preparation, analysis, and reporting. It enhances human analysis with AI-driven automation across key stages.
Gartner defines augmented analytics as:
“Enabling technologies like machine learning/AI that assist with data preparation, insight generation and insight explanation to augment how people explore and analyze data in analytics and BI platforms.”
In other words, it strategically complements human intelligence around data with the computational power of machines – for faster discovery of deeper insights without needing coding skills or data science expertise.
The Core Capabilities:
- Data Identification: Automatically recognizing data attributes and entities for appropriate handling by learning context over time.
- Data Preparation: Cleaning, structuring, and formatting data rapidly by suggesting relevant steps driven by internal algorithms.
- Insights Generation: Highlighting key trends, correlations, outliers, etc, via statistical modeling and machine learning techniques on processed datasets.
- Natural Language Interactions: Allowing search, query, and reporting through conversational inputs that get translated into appropriate technical commands internally by the system.
- Visualization Recommendations: Proposing apt chart types, color schemes, and layouts tailored to selected data fields and metrics using internal logic.
Why Augmented Analytics Matters?
Here is why augmented analytics matters:
Democratizing Data Insights Through Augmented Intelligence
Augmented analytics integrates machine learning into business intelligence platforms – assisting users across key analytical workflow stages while expanding access to impactful data insights across the enterprise.
By automating complex, repetitive tasks and surfacing contextual recommendations, augmented analytics aims to simplify usage while unlocking wider value from data assets – accelerating the journey toward becoming an insights-driven organization.
Empowering Deeper Data Discovery
Augmented analytics democratizes access to advanced analytics at scale by empowering functional roles beyond technical teams to unlock deeper insights autonomously. The integration of AI-driven automation assists non-technical users across business units in preparing, analyzing, and visualizing data more efficiently – acting on findings that drive impact.
Rather than competing with technical talent, it augments them by simplifying complex tasks so they can focus on higher-value work. This provides a scalable solution to the data talent shortage that plagues most enterprises, even while making analytical thinking open to more teams through automation.
Accelerating Speed to Insight
The rapid experimentation and analysis cycles enabled by machine learning algorithms help uncover valuable insights faster than manual approaches. Augmented analytics harnesses the computational power of models to reveal trends, outliers, and signals within massive and multivariate data sets that humans working solo may miss or only detect after tedious exploration.
It shortens the iteration loop, allowing users to ask more questions and gain more answers from their data assets quickly. The pace of informed decision-making thus accelerates enterprise-wide. Rather than getting stuck analyzing what happened, there’s an opportunity to predict and prescribe what should happen next.
Enhancing Personalization
Based on monitoring interaction patterns and querying behaviors over time, augmented analytics platforms can provide tailored suggestions and experiences that are smarter, more relevant, and resonant for each individual user.
As adoption progresses across customer-facing and operational roles, these personalized recommendations enhance both internal team productivity as well as external customer experiences – driving efficiency alongside satisfaction through individualization at scale.
Lowering Adoption Barriers
Conversational, interactive interfaces powered by AI make working with data approachable for less technically savvy employees, while visual-first reporting lowers barriers to conveying analytics stories powerfully.
Augmented analytics solutions demystify coding complexity while assisting users in articulating and acting upon data insights using natural language – enabling an informed, analytical culture to permeate top-down more swiftly, even with variability in existing expertise. The focus is getting the right insights to the right people at the right time using the right interface.
In summary, by using AI techniques to enhance human intelligence around data, augmented analytics solutions aim to make organizations fundamentally insights-driven. The mandate is to assist leaders, analysts, and operators in discovering signals faster, personalizing experiences smarter, and driving actions bolder by democratizing access to data and upgrading skills for all. Future enterprises will thrive on augmented intelligence.
Practical Enterprise Applications
Augmented analytics strategically integrates machine learning into business intelligence platforms to assist users across critical analytical workflow stages – while expanding access to impactful data insights across the enterprise.
By automating complex, repetitive tasks and providing contextual recommendations, augmented analytics aims to simplify usage while unlocking wider value from data assets. This accelerates the journey towards becoming an insights-driven organization.
Let’s explore how five core business functions can derive competitive advantage from augmented intelligence:
Enhancing Customer Experiences
By combining transaction history, web analytics, demographics, and external signals, augmented analytics can swiftly reveal customer lifetime value projections, churn risks, cross-sell opportunities, and micro-segmentation profiles – insights human analysts would likely miss.
This powers hyper-personalized marketing and sales plays while optimizing resource allocation at scale using machine intelligence to crunch big data sets rapidly. Augmented analytics uncovers the drivers that truly influence customer satisfaction and loyalty.
Optimizing Supply Chain Operations
Analyzing purchase records, shipment data, warehouse inventories, supplier ratings, and even reviewing weather forecasts allows intelligent algorithms to rapidly highlight bottlenecks, quality issues, demand-supply gaps, and logistics performance factors.
By proactively flagging risks ahead for procurement, manufacturing, and distribution teams, augmented analytics enables data-informed decisions on production planning, inventory policies, and network resilience strategies. The supply chain becomes intelligent, predictive, and prepared.
Informing Growth Strategies
Processing historical financial statements, budgets, economic indicators, market variables, and even news trends helps advanced AI models uncover seasonal revenue patterns, predict earnings shortfalls, simulate budget scenarios, and estimate the impact of strategic investments or macro-events.
Augmented analytics provides the analytical foundation for shaping corporate development priorities, capital allocation plans, and managing operating costs in an agile fashion – assisting executives in steering towards growth.
Advancing Healthcare Delivery
Patient health records, clinical diagnostics, medical research journals, and population health data contain crucial interrelationships that algorithms can effectively decipher to boost diagnostic accuracy, personalize treatment pathways, pragmatically monitor outcomes, and model disease progression scenarios.
By revealing individual health trajectories, augmented analytics paves the way for preventive, predictive, and precision-based medicine – helping save and improve more lives. It also assists policymakers and administrators in shaping practical programs aligned with community needs based on empirical evidence.
Innovating Manufacturing Processes
By interfacing assembly line sensors with enterprise data sets on product component specs, machine operating procedures, warranty benchmarks, and even employee shift timings, manufacturers can rapidly gain AI-generated insights on production yield risks, process bottlenecks, predictive maintenance needs, safety stock levels, and factory energy optimization.
Augmented analytics provides real-time visibility that drives significant quality, cost, and productivity gains – accelerating the future of smart manufacturing.
In summary, across key functions, augmented analytics can guide enhanced decision-making to help enterprises gain sustainable competitive advantage through data-driven, fact-based planning focused on delighting customers profitably.
These are just some examples of data-driven transformation through augmented intelligence across domains, functions, and use cases, enabling competitive advantage. Now, let’s explore the top tools in this space.
Key Augmented Analytics Tools
Here are some augmented analytics tools:
1. Tableau
Tableau augments analysis through features like Ask Data, Explain Data, and AI-driven insight recommendations using the vendor’s proprietary Einstein Discovery capability. Enables easy adoption across user segments.
- Ask Data: Allows users to query data just by typing questions in natural language. It then translates the questions into appropriate data visualizations.
- Explain Data: Automatically generates textual descriptions and summaries to explain key trends and insights from data visualizations. Makes discoveries easy to understand.
- Einstein Discovery: A proprietary machine learning-powered capability to automatically discover and visualize insights, identify key factors driving outcomes, and make predictions. Requires no data science expertise.
These features enable users across different skill levels to easily adopt Tableau for smart data analysis and storytelling, accelerating the path to becoming insights-driven.
2. Microsoft Power BI
Power BI now incorporates Azure Machine Learning models to enhance analytics through natural language queries, auto-generated visuals, and predictive forecasts using external datasets.
- Natural Language Queries: Users can ask questions about their data in plain language instead of writing queries and receive automated visualizations in response.
- Auto-generated Visuals: The system can automatically create appropriate basic data visualizations with minimal inputs based on the data fields selected.
- Predictive Forecasting: Inbuilt algorithms can process historical data to deliver user-friendly predictive insights about future outcomes without the need for coding.
By democratizing access to advanced analytics, Power BI simplifies data-driven decision-making across organizations.
3. Qlik
The Qlik platform offers augmented analytics through its cognitive engine for NLP-based interactions and insight suggestions powered by associated algorithms. Also supports embeddings for predictive modeling.
Key augmented capabilities offered by Qlik include:
- Cognitive Engine: The vendor’s proprietary AI capability for natural language interactions, conversational analytics, and smart visualization recommendations.
- Insight Advisor: An automated statistical assistant that suggests relevant insights, trends, and associations within large data sets without requiring technical expertise.
- Embedding Analytics: Allows predictive analytics and machine learning model outputs to be easily embedded and consumed across any application through APIs.
With its strong analytics heritage spanning decades, Qlik offers a robust enterprise-class augmented analytics solution.
4. Sisense
The Sisense platform provides AI assistance for rapid data preparation and modeling, while an Insights Assistant offers statistical analysis automation and forecasting. Low-code options ease usage.
Sisense provides augmented support across the analysis workflow:
- AI Data Preparation: Automates complex data integration tasks in a unified interface, reducing manual overheads.
- Insights Assistant: Offers statisticians, data scientists, and business users automated insights and forecasts to accelerate discovery.
- AI Models: Allows data teams to develop, operationalize, and monitor custom machine learning models through a no-code model builder interface, democratizing access.
Sisense makes cutting-edge data science accessible to users of all skill levels – accelerating innovation.
5. Yellowfin
This end-to-end analytics platform features automated data preparation, NLQ query assistance, and predictive workflows integrated across modules. The vendor-agnostic solution also supports data storytelling. Yellowfin focuses on automating insights discovery:
- Automated Data Prep: Machine learning speeds up data integration, cleaning, and shaping with recommendations.
- Natural Language Generation: Automates simple narrative descriptions and highlights from dashboards and visualizations.
- Predictive Analytics: Embedded automated forecasting, clustering, and association rule mining techniques need no coding.
The emphasis is on collaborative analytics and automated storytelling capabilities that cater to business users through flexible pricing tiers.
As this list indicates, most leading enterprise BI tools now incorporate some degree of augmented analytics capabilities natively coupled with options to integrate complementary third-party services as needed. The stacks continue to advance rapidly.
However, simply adopting the appropriate tools alone rarely guarantees analytics success. The degree to which organizations can draw value is directly linked to mitigating associated adoption challenges – both technological and cultural.
Overcoming Top Adoption Barriers
Transitioning workflows, metrics, and stakeholders accustomed to static reporting dashboards to adopt more advanced analytics inevitably encounter hurdles – some unique to augmented techniques. Being aware helps accelerate appropriate strategies. Let’s discuss a few key challenges:
Data Quality
As analytics gets increasingly automated through algorithms dependent on available data, incomplete or dated datasets severely inhibit the reliability of machine-generated insights. Maintaining data integrity and security thus becomes foundational.
Trust in AI
Black box analytics contradicts augmented analytics objectives. Outputs seem arbitrary without explanations concerning the reasoning and data-driving decisions. Ensuring transparency by tracking model lineage from input to output stages cultivates user trust in adopted solutions.
Collaborative Workflows
Custom models built by central data teams often operate in isolation. Facilitating integration of augmented analytics outputs into familiar dashboards, reports, and applications used enterprise-wide maximizes business impact through natural adoption reinforcement.
Role Alignment
The proliferation of citizen data science capabilities requires reassessing roles. Rather than competing with technical teams, establishing clear lanes for business units to leverage automation in solving domain problems provides clarity. Data scientists transition to being stewards.
Platform Governance
As usage scales, controlling model sprawl and tool multiplicity through centralized model management, monitoring, and ops becomes necessary – along with instituting robust access protocols aligned to data sensitivity.
Addressing these factors holistically sets the stage for broader assimilation of intelligent analytics – allowing humans augmented by machines to thrive together in extracting hidden insights from data.
The Future State
In 2019, Gartner predicted that by 2020, over 50% of analytics queries will be generated using NLP or automated means as augmented analytics pervades business workflows. As innovative techniques get democratized for productivity gains without demanding advanced data science skills, investments will continue rising.
Forrester notes that early adopters have already observed employees saving over 50% of time previously spent in preparing and analyzing data after adopting augmented analytics capabilities – clearly indicating ROI potential apart from deeper insights unlocked.
As enhanced machine learning lifts barriers to insight discovery through automation, humans get empowered to ask better questions and make decisions quickly. But humans must remain at the center – directing purpose, adding context, and determining action. Augmented analytics aims to support, not replace.
The future state will see humans empowered with an augmented intelligence spanning both their individual potential and the collective knowledge captured by machines from data – enabling the power to gain deeper insights faster from any device. That future awaits.
Conclusion
In conclusion, augmented analytics integrates machine learning and AI into business intelligence platforms to assist users across key analytical workflow stages. It expands access to impactful data insights across organizations by automating complex tasks and providing contextual recommendations.
Augmented analytics solutions aim to simplify usage while unlocking wider value from data assets, helping companies accelerate their journey toward becoming insights-driven. As seen through real-world examples, leading tools like Tableau, Microsoft Power BI, Qlik, and more incorporate robust capabilities for natural language interactions, smart visualization, and predictive modeling – all easy enough for citizen data scientists.
To gain hands-on experience with augmented analytics yourself, check out CCS Learning Academy’s comprehensive analytics and BI courses covering everything from data visualization to developing AI/ML models.
FAQs
Augmented analytics is an approach that uses machine learning and artificial intelligence techniques to automate data preparation, insight generation, and insight explanation. This enables a deeper, more democratized use of analytics across business users, operational workers, and data scientists alike
Augmented analytics is important because it enhances decision-making by providing faster and more accurate analyses. It reduces the manual effort traditionally associated with data science, such as data preparation and model selection, making advanced data analysis more accessible to non-experts and enhancing productivity.
Common features include automated data preparation, natural language processing for generating narrative insights, anomaly detection, and predictive analytics. These features help streamline the analysis process and make complex data insights more accessible to a broader audience.
Examples of augmented analytics include a marketing team using it to predict customer behaviors, a finance department automating fraud detection, and HR leveraging it to analyze employee turnover rates. Augmented analytics can provide predictive insights and recommended actions, enabling proactive decision-making across various business functions.
Tools that support augmented analytics include Tableau, which integrates advanced analytics into visualizations, Microsoft Power BI with AI capabilities, Salesforce Einstein for CRM-related insights, and Oracle Analytics Cloud, designed to automate insights using AI.
Unlike traditional BI, which relies heavily on manual data analysis and report generation, augmented analytics uses AI and ML to automate these processes, providing insights more quickly and with less human intervention. This not only speeds up the analytical process but also enables more complex analyses that are typically beyond the reach of manual methods.
While augmented analytics tools are designed to be user-friendly and accessible to non-specialists, a basic understanding of data analysis concepts and familiarity with the specific tools are beneficial. Skills in interpreting data visualizations and making data-driven decisions are also important.
Benefits of using augmented analytics include increased efficiency in data analysis processes, reduced bias and errors through automation, enhanced data literacy among business users, and the ability to uncover deeper insights through advanced analytical techniques.
Challenges can include the initial cost and complexity of integrating new systems, the need for cultural change within organizations to adopt data-driven decision-making, and potential data privacy and security concerns when using automated systems.
Future trends include the increasing integration of AI and machine learning capabilities directly into business applications, greater use of natural language processing for generating and interpreting analytics, and the continued democratization of data analytics, allowing more end-users to perform complex data analyses without deep technical knowledge.