10 Types of Data Visualizations You Must Know in 2024
- -
- Time -
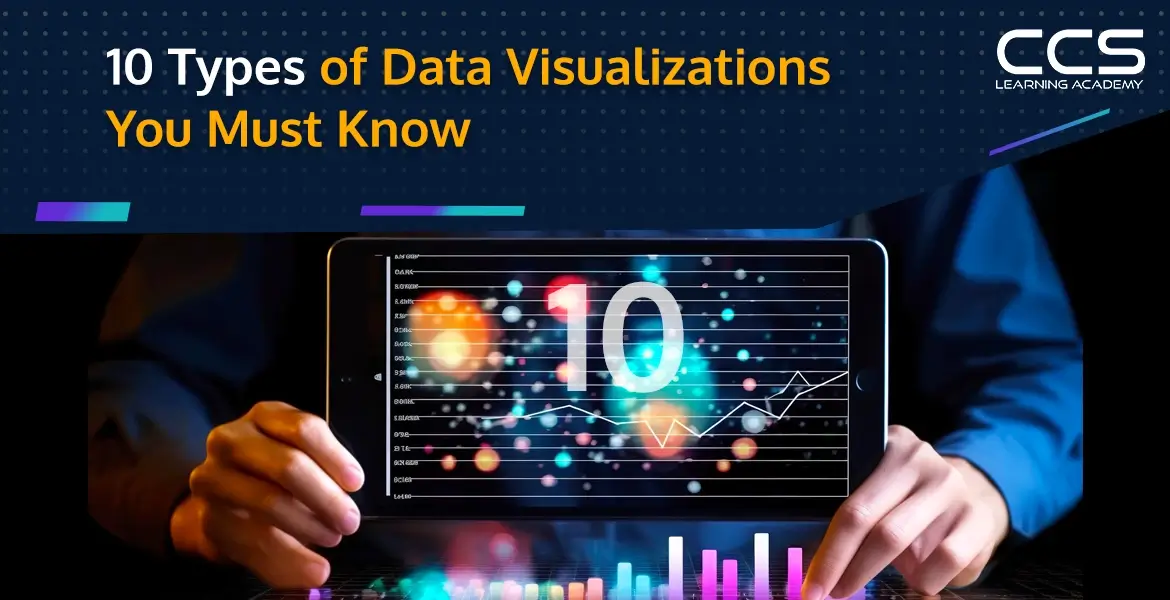
Leveraging visualizations to present complex insights simply and clearly is an indispensable skill across industries. The right graphs and charts spotlight trends, outliers, and patterns buried within the numbers to tell simplified yet high-impact data stories.
With an expanding analytical toolbox, understanding the strengths of fundamental as well as novel visual types matching analysis needs is key to deriving meaning effectively. This guide will explore the top 10 data visualizations that every analyst should have command over by 2024 to spearhead data fluency powering smarter decisions.
What is Data Visualization?
Data visualization refers to the graphical representation of information and data. It involves creating visual depictions like charts, graphs, and maps to give viewers a clear overview of complex data sets, enabling them to identify patterns, trends, and outliers more easily.
At its core, data visualization transforms numbers and facts into visuals that make complex concepts and insights more accessible to a wider audience. It allows everyone from analytics leaders to frontline employees with no technical expertise to quickly grasp key findings from data analysis. This drives better, faster decisions aligned to data across teams and stakeholders.
Some key benefits of data visualization include:
- Highlights insights that may otherwise get lost in rows and columns of raw data
- Condenses large data sets into easily interpreted visuals
- Improves comprehension of analytics and conclusions
- Enables quicker identification of relationships and patterns
- Appeals to visual learners with graphic representations
- Captures attention and interest better than plain text
How to Select the Appropriate Graph or Chart
When choosing data visualizations, using the right chart type is critical to accurately represent what the data means. This requires aligning attributes of the visualization with the specifics and objectives of the data. Key considerations for optimal chart selection include:
Purpose
Be clear on what the data visualization aims to achieve – is it projecting trends or correlations? Showcasing compositions and parts of a whole? Demonstrating changes over time? Benchmarking performance across indicators? Defining purpose guides appropriate chart choice. A visualization must serve its analytical goal foremost before aesthetics.
Data Types
The data variables drive what graphic suits it best. Quantitative, time-series, and spatial data do better with specific chart types based on their characteristics. Knowing the data properties and formats enables matching it with the right visualization fit.
Target Audience
The consumer context guides design – their analytical maturity and data literacy determine optimal complexity. A visualization for senior executives focuses on key summaries while analysts require richer multidimensionality. Tailor visual displays to audience needs.
Visual Style and Patterns
Maintaining visual consistency, clean designs, and intuitive data mapping that aligns with viewer intuitive expectations enhances interpretations and memorability which builds data fluency across teams when applying similar data visualization grammars repeatedly.
By consciously aligning core visualization aspects with key data attributes and intended goals, analysts derive richer meaning and impact from data representations. This declarative approach to information design propels fact-based communication and drives optimal data-driven decision-making across enterprises.
10 Types of Data Visualizations You Must Know in 2024
Here are the types of data visualizations in 2024:
1. Bar Charts
Among the most common yet versatile data visualization options, bar charts use rectangular bars with variable lengths proportional to the data values they represent. The bars can illustrate comparisons across qualitative categories and quantitative measurements effectively.
Key Features:
- Displays rank orders through bar heights
- Conveys part-to-whole relationships
- Easy comparison of amounts between classes
- Handles both nominal and numerical data
Best Applications:
- Compare amounts across categories like sales variances across regions, revenue contribution of products, market share standings, etc. over time
- Display hierarchical structures and stacks illuminating compositions like workforce demographics segmented further
- Highlight before-after differences resulting from intervention through grouped bars
Bar charts simplify relating quantities and degrees through length comparisons rather than numeric accuracy. However, they lose impact when categories exceed 10. Horizontal bar charts allow higher label readability where category names are lengthy by transposing axes. Grouped bars enable intra-class evaluations.
2. Line Charts
Among the most flexible options, line charts display continuous data evolving over intervals through sequences of connected data points called markers. The intuitiveness of direction and slope trends drives interpretation and forecasting.
Key Features:
- Tracks patterns efficiently over time/spectrum
- Highlights trajectory and projectivity clearly
- Compares multiple time-series together
Top Applications:
- View trends of key business metrics like revenue, costs, and foot traffic over years/months
- Monitor machine sensor data, stock prices, humidity, and temperature over time
- Overlay multiple indicators’ historical movements together highlighting correlations like sales and marketing expense tracking
Lines efficiently compress progression and variance data across many time points into easily traced summary trajectories. Dotted lines help compare predictions vs actuals. However, too many lines risk indiscernible clutter. Pairing with other types like bar charts clarifies relationships.
3. Scatter Plots
Scatter plots depict the correlation between two numeric variables by plotting data points at axis intersections matching values along dimensions. Instead of trends, they showcase linkage strengths, deviations, and anomalies.
Characteristics:
- Each data point conveys paired values
- Distance from fit conveys variability
- Direction, shape, and proximity show relationships
Scenarios:
- Relate pricing to sales volumes and identify value sweet spots
- Map age and income clusters to target segmentation
- Overlay production input factors against output efficiency identifying input optimization
The distribution and scattering of points reveal covariance patterns better than statistics like R-squared alone. Heat map shading the background by density adds another variable injection. Scatter plots help benchmark practices evaluating performance impact across change levers through factor comparisons.
4. Pie Charts
A popular option, pie charts represent categorical proportions converting metrics into percentage slices of a whole pie in a part-to-whole structure through angled wedges. Their circular completeness and additive property simplify data relationships.
Characteristics:
- Conveys part-whole visual parallels
- Highlights proportionality and comparison
- Captures categorical compositions
Use Cases:
- Breakdown of sales contribution of products/services
- Contrast departmental budgets/spending
- Segment audiences by behavioral attributes like demographics
Pies effectively showcase compositions and variance across components in a synergized way. Exploded pies help pull out select slices selectively for emphasis. Limit categories below 6 with clearly different color codes for discernible separation. They can suffer from less accuracy in conveying quantities visually over simple stats.
5. Heat Maps
Heat maps visualize two-dimensional data where color intensity depicts concentrations of intersection values between attributes with higher color depth representing larger values via a gradient color legend. They best convey densities across continuums.
Key Features:
- Color-coded patterns help spot correlations
- Data clustering stands out
- Scalable across data sizes for the big picture
Top Applications:
- Digital visit/click frequency across website pages
- Store heat maps of purchases across geographic locales
- Demand forecasting seasonal variability
The color depth generated makes hidden insights like purchase confluences, traffic trends, and clustering patterns easily perceptible. However, heat map efficacy reduces with more attributes and overlaps. Paired with scatter plots, the combination enables granular visualization. For sequential data, tree maps add richer detail.
6. Histogram
Histograms visualize the distribution of continuous data values across uniform intervals, displaying the frequency of data points within predefined buckets through adjacent bars representing each range cluster. They illuminate concentration and anomalies.
Characteristics:
- Reveals data distribution and frequencies
- Identifies irregularities and outliers
- Compares multiple distributions
Scenarios:
- Contrast monthly sales volume frequency
- Gauge sentiment intensity across customer reviews
- Benchmark process quality range deviations
The interval-based aggregation provides discretion around irregular densities, unlike time-bound line charts. Histograms help assess central tendency proximity, variability, and shapes illuminating data qualities and field factors for informed statistical modeling. Placing comparative histograms provides fast outlier diagnoses. But interval setting biases interpretation.
7. Radar Charts
Also known as spider charts, radar plots showcase multivariate data spanning quantitative metrics and performance dimensions using axis spokes from a common center point with data lines connected across values conveying rich qualitative insights.
Key Features:
- Handles multivariate complex data
- Reveals performance gaps precisely
- Compares rated capabilities better
Top Applications:
- Product capability benchmarking
- Employee competency diagnostics
- Hotel service quality analysis
The 360-degree visibility improves contextual evaluations across pertinent facets unlike limiting data slicing. Radar plots help assess group progressions over time and showcase operational diagnostics concisely helping multi-criteria decision analysis. But variable ordering biases interpretation and overlapping lines muddle insights when data sets grow bigger.
8. Tree Maps
Tree maps display hierarchical data by recursively partitioning attributes into rectangular tiles using a nested variation of size, color, and density mirroring structures like org distribution, product categorizations, etc with the entire tile area proportionally representing a whole quantity subdivided into smaller constituent tiles by factors.
Key Characteristics:
- Reveals both composition and quantities
- Highlights hierarchies and asks focally
- Handles multivariate complex data
Use Cases:
- Analyze sales portfolio product mixes
- Contrast demographics spread
- Diagnose manufacturing defect densities
Tree maps enable intuitive data exploration across granular and organization levels with their nesting ability to provide textured visibility impossible otherwise. Their space optimization handles multivariate data well. However, navigating dense tessellation complexity poses challenges in data identification.
9. Waterfall Charts
Waterfall charts detail sequential positive or negative changes between intervals through cascading vertical pillars summing up transparently. Each color-coded column denotes delta movement highlighting contributors and inhibitors behind endpoint deviations and variance forensics.
Key Features:
- Pinpoints incremental differences
- Quantifies sequential variations impactfully
- Handles multivariate sources well
Top Applications:
- Analyzing sales growth contributing factors
- Forecasting cash flows by modeling assumptions
- Diagnosing patient pathway process efficiencies
Waterfall clarity around multi-tiered drivers helps target operational improvement priorities better. They work well for financial statement analysis and variant optimization use cases. But their signal isolation simplifies system interrelationships. Pairing predictive modeling helps offset risks.
10. Sankey Diagrams
Sankey charts visualize interconnected structural flows and multi-dimensional transitions and magnitudes within network topologies. The path thicknesses across nodes graphically represent the movement weights and volumes highlighting critical transfer points for systematic efficiency improvements.
Key Characteristics:
- Spot constraint points in systems
- Track multidirectional network flows
- Benchmark network performances
Scenarios:
- Examine manufacturing production system flows
- Analyze website visitor clickstreams funnels
- Survey talent pipeline workforce dynamics
Sankeys inject network science perspectives into process system analysis revealing friction points for smoothing operations, conversion risks, and deviational diagnosis offering process playbooks enhancing experiences. But growing complex links exponentially complicates insights generation needing selective pruning for clarity.
Making Data Speak
The growing rationalization of decision-making requires framing information and ideas visually to create sticky stories and memorable models that steer choices and strategy. Each data visualization format serves specific analytical needs better. Prioritizing options by matched utility ensures extracting meaning more effectively rather than force-fitting.
While trending options like augmented charts keep emerging, foundational literacy across established and novel types is indispensable for any leading practitioner to expand their data science repertoire to structure thinking shaped by perspectives revealed through the simplest yet impactful visualization facilitating discoveries hidden inside the numbers waiting to be unveiled.
Those who master the lexicon of charts along with discerning the art of contextually applying them access a versatile toolkit elevating the way they make sense of the world helping pioneers pull the future forward utilizing these visual instruments of thought-empowering ideas into actions one insight at a time while making data speak volumes!
Conclusion
With growing data volumes across operations and touchpoints, leveraging intuitive visualizations offering clarity and direction becomes pivotal rather than simple reporting alone. This overview of the top 10 data visualization types that every analyst should know by 2024 covers fundamental as well as novel representations across best practice use cases spanning statistical analysis, performance evaluations, and process diagnostics needs among other scenarios.
Beyond just employing tools, deliberately building fluency across foundational visualization grammar is crucial. When matched with needs, this accelerates analytical competencies for business analysts and data scientists. This approach cements data-driven perspectives, which are central to strategic roles driving enterprise growth amid mounting market variability and opportunities.
Aspiring leaders investing in continual learning across heuristic and pragmatic approaches positioning data visualizations as trusted advisors unlock lasting value.
CCS Learning Academy offers a Data Analytics & Engineering Bootcamp certificate that you can earn in just 12 weeks with dedicated 1-1 mentorship. This program will cement foundational data fluency, accelerating analytical competencies through a curriculum spanning statistical analysis, visualization best practices, and hands-on tools application – unlocking career growth opportunities in one of the highest-demand domains today.
FAQs
There are various types of data visualizations, including bar charts, line graphs, scatter plots, pie charts, area charts, histograms, heatmaps, bubble charts, box plots, and radar charts.
A bar chart is a visual representation of data using rectangular bars, where the length of each bar corresponds to the value it represents. It is commonly used to compare categorical data and show trends over time.
A line graph displays data points connected by lines, making it ideal for showing trends and changes over time. It is often used to visualize continuous data, such as stock prices, temperature variations, or sales trends.
A scatter plot is used to visualize the relationship between two variables by plotting data points on a two-dimensional graph. It helps identify patterns, correlations, and outliers in the data.
A pie chart is used to represent the proportions or percentages of a whole. It is suitable for displaying data with a few categories and comparing their relative sizes.
An area chart is similar to a line graph but fills the area under the line, making it suitable for visualizing cumulative data or stacked proportions over time. It helps show the total value as well as the contribution of each category.
A histogram is a graphical representation of the distribution of numerical data, showing the frequency of values within specific intervals (bins). It helps visualize the shape, center, and spread of the data distribution.
A heatmap is used to visualize data in a matrix format, where colors represent the magnitude of values. It is often used to identify patterns, correlations, and clusters in large datasets, especially in fields like finance, biology, and sports analytics.
A bubble chart displays data points as bubbles, where the size of each bubble represents a third variable. It helps visualize relationships between three variables and identify patterns or trends within the data.
A box plot, also known as a box-and-whisker plot, is used to visualize the distribution, spread, and skewness of numerical data. It indicates the median, quartiles, range, and potential outliers in the dataset.