Best Data Analysis Methods: Choose the Right Technique
- -
- Time -
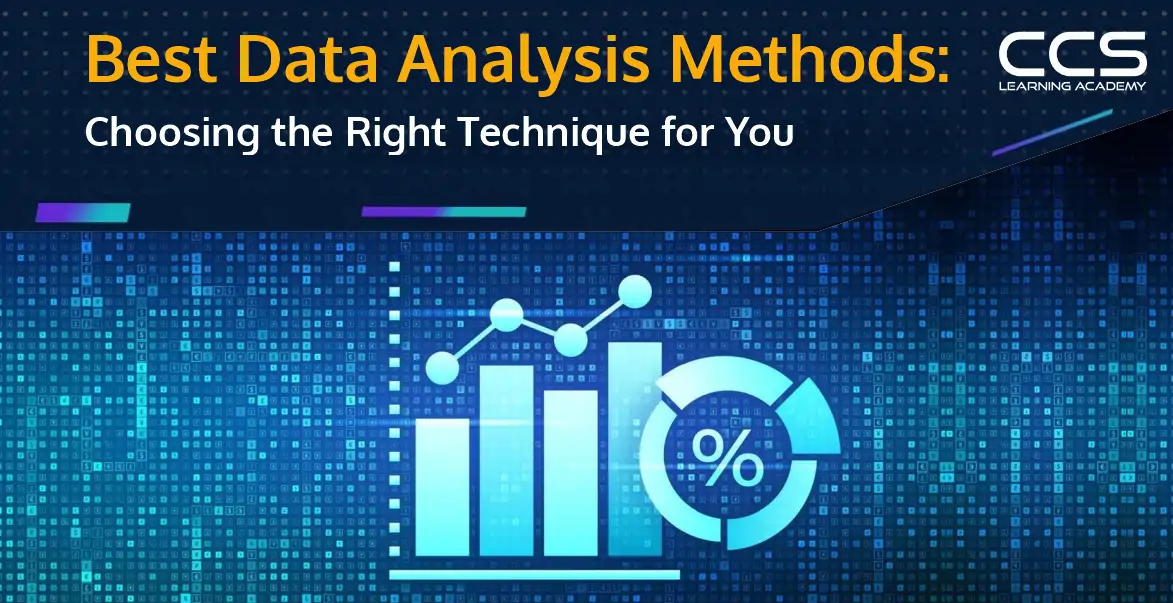
Data analysis is the process of transforming raw data into actionable insights for informed decision-making. There are various data analysis methods that people use to generate valuable insights.
According to the US Bureau of Labour Statistics, the operations and market research data analysis will likely grow by 25% and 18%, respectively. Many professionals are joining Data Analytics & Engineering Bootcamp to get certified, gain knowledge and get professional growth.
Well, to incorporate this into your business or research, learn what are the data analysis methods and how to choose one to obtain your goals, then this blog is for you. Read research methods & data analysis for multicultural social work and human services online free and find the opportunity to develop your expertise in your field.
How Data Analysis Is Used In Business?
- Data analysis turns raw data into actionable insights by inspecting, cleaning, and transforming it to support decision-making.
- It helps businesses make informed decisions in marketing, investment, and budgeting.
- Data analytics involves collecting, cleaning, and modeling data to reveal trends, patterns, and insights, guiding leaders in making data-driven decisions and improving efficiency.
- Market research commonly uses it to track customer engagement and forecast future trends.
Most Effective Data Analysis Methods
To analyse data and draw conclusions, you don’t need to be a data analyst or have a Ph.D. Various practical data analysis techniques are very simple to employ. Let’s have a look at all these methods in detail.

1. Descriptive Data Analysis
This method is considered the beginning point of an analytical journey. This type of technique follows ordering factors that manipulate and interpret varied data from multiple sources and then turn those data into valuable insights.
In addition to this, conducting this analysis is significant as it allows you to showcase your derived information in a streamlined method.
Advantages | Limitation |
---|---|
Maintains a high degree of objectivity and neutrality. | Descriptive analysis process is time-consuming and often requires substantial efforts. |
Provide accurate representation of data. | Cannot be utilised to make predictions based on given data values. |
Helps in hypothesis generation and variable identification. | Inability to forecast future scenarios and changes. |
Tracks key performance indicators like sales, revenue, retention, and loyalty. | Improper execution of analysis may result in misleading conclusions. |
Offers flexibility in data utilization and efficient data collection. | Lacks strong connection with other data points. |
Applicability
- Sales performance: A retail company uses these statistical methods for data analysis to understand the average sales volume per store and find which products are their best-selling.
- Customer satisfaction surveys: Companies use this method to analyse survey data and find the most common customer responses.
2. Qualitative Data Analysis
This type of technique cannot be measured directly, and thus, it is used to make decisions based on subjective interpretation. Organisations often use qualitative data analysis methods to evaluate customer feedback, get survey insights, analyse specific changes, and determine the effectiveness of social media posts or features of a product.
The primary objective of this technique should be to identify meaningful insights or to obtain answers from unstructured data like vocal feedback, transcripts, and many more. Furthermore, qualitative analysis aids in organizing data into different categories for further automation.
Advantages | Limitation |
---|---|
Allows you to analyze underlying motivation, attitudes and behavior in-depth. | The data sample size can be an issue. |
Examine complex issues and relationships. | Different biased opinions of the people in the sample group. |
Adds context and explains the insights that numbers cannot reveal alone. | Inability to verify the results of qualitative research. |
Provides real interaction to uncover valuable insights. | Follows a labour intensive approach. |
Applicability
- Market analysis: A business may analyse the product’s sales and its journey. It helps in the identification of sales and where it has spiked up in a particular quarter. It shows price changes and market trends.
- Medical diagnosis: Clinicians and various medical researchers use this method to understand the cause of symptoms based on lab results and patient data.
3. Quantitative Analysis
Quantitative analysis refers to measurable information that involves specific numbers and quantities. The core strength of quantitative data analysis methods is that they use mathematical and statistical techniques to analyse numerical data. This type of method examines numeric data to identify patterns and quantify relationships between them.
Advantages | Limitations |
---|---|
The method is more objective and is not subjected to any biases. | It does not include analysis of non numerical data like images, audios etc. |
It analyses data over a larger sample size covering various data sets. | It does not say the full analysis as it is limited to just numerical data. |
This method provides an analysis faster and is easier to collect data. | The method overlooks broader themes and relationships among various stakeholders and thus the findings can be inconclusive. |
It gives a statistical analysis and suggests which statistical tests to employ. | Is not a great tool to get data if focus is on getting non numerical analysis. |
Applicability
- Budget estimation: Allows investigation of the correlation between advertising expenses and sales revenue.
- Financial and investment decisions: A financial analyst uses quantitative analysis to evaluate the stock performances and calculates the various financial ratios using statistical tests.
- Email Campaigning: It is also used for email campaigning, like click baits, in the estimation of sales figures, website visitors, employee performance, and revenue generation.
4. Predictive Data Analysis
It enables you to answer the “what happens next” type of questions. You can combine the results obtained from this analysis with machine learning and artificial intelligence to identify potential issues or loopholes in your dataset. Also, it helps in further discovery and development of insights.
With easy-to-understand information, businesses can find trends and create common patterns, making valuable initiatives or decisions for further operations. This type of analysis involves the utilisation of historical data to predict future outcomes.
Advantages | Limitations |
---|---|
Enables businesses to make accurate decision making. | If available data is incomplete, outdated, or biased, it affects the performance of this analysis. |
Helps in future planning and risk mitigation for long term success of business. | Complex machine learning algorithms make it difficult to interpret. |
Improves customer insights and personalization. | It is based on past data, and assumes future scenarios will depend on those patterns and relationships. It ignores the changing dynamics of the market. |
Offers cost-effective approach. | |
Gives businesses a competitive advantage to stay ahead of market trends. |
Applicability
- Credit scoring: Various financial institutions use predictive models to assess a customer’s availability to attempt a defaulting loan.
- Weather forecasting: Meteorologists use this predictive data analysis to forecast weather conditions based on past data.
- Examples: Forecasting stock prices, predicting customer buying behaviour, and sales revenue.
Read more: How to Use AI in Data Analytics: Ultimate Guide
5. Diagnostic Data Analysis
Diagnostic data analysis can help you identify why your sales are declining and allows you to explore the factors affecting its loss.
This technique offers actionable answers to your specific questions of “how it happened”. It is one of the most preferred methods of data analysis in research and varied domains.
Advantages | Limitations |
---|---|
It provides deeper insights in data when used with other analytics tools. | It often confuses correlation for causation while comparing two different items in data. |
The method helps in detecting anomalies in the data. | It is not a predictive tool and cannot tell what the future may look like based on current data. It only reports what has happened in the past. |
It helps you in identifying mistakes and helping you improve it to become more efficient in the future. | The findings of diagnostic analysis must be coupled with analysis of predictive analysis to know what needs to be done in the future. |
The visualization of data is easy to understand. | It does not provide definitive answers and only tells the percentage of occurrence of any event that may have been caused by the interaction of a certain percentage of objects or people with any event. |
Applicability
- Sales and customer support: Investigations of sudden spikes in product sales can be done using diagnostic analysis. Customer complaints cause, patterns, and anomalies are identified using this analysis.
- Inventory analysis: Diagnostic data analysis allows you to check if fewer sales correlate with stock outs or overstock situations.
- Effective promotion: Allows businesses to analyse the impact of different promotional campaigns to see what concepts failed to attract customers.
6. Regression Analysis
The method utilises historical data to understand the impact of the dependent variable’s value. This type of analysis is a foundational statistical method used to model and analyse different relationships between variables. Furthermore, it estimates how other variables influence one variable.
The primary goal of such basic data analysis methods is to predict and explain. It forecasts the outcomes based on identified relationships and influences the predictor variables. Furthermore, regression relies on several assumptions and observations to derive better results.
For example, if you conduct a regression analysis on your sales report and the results represent factors like customer services, sales channels, marketing campaigns, etc., you can conduct another regression analysis to check if the variables changed over time or if new variables are now impacting your sales results.
Advantages | Limitations |
---|---|
It can indicate which consumer group has contributed to the business growth. | It is a costly process and The regression analysis method is used for short analysis to examine two types of email campaigns, known as A/B testing over time. This enables you to understand which variation has turned out to be responsive for you in terms of time and timely data updates for accuracy. |
Helps get key insights into customer behavior. | It can lead to ethical problems in health studies. |
Enables you to have a targeted marketing strategy by helping in customer segmentation. | It is not efficient when working with rare diseases or a small sample size. |
Enables you to have a targeted marketing strategy by helping in customer segmentation. | It is not efficient when working with rare diseases or a small sample size. |
Identify potential churn risks and that helps businesses identify retention rates. | The data is prone to fluctuations in it’s further updates. |
Applicability
- It can be used in versatile fields like business, economics, biology, and healthcare.
- Market trend assessment: Evaluates how market changes in economic environments and also identifies issues in sales reports for businesses.
- Predictive pricing: Using historical data allows you to predict future price trends depending on the current market dynamics.

7. Cohort Analysis
This analysis includes the study of historical data for further analysis of specific segments in user behaviour and groups. Cohort analysis techniques can provide an idea of your customer’s and target audience’s needs. You can use this analysis to determine the impact of a marketing campaign on the targeted audience.
Advantages | Limitation |
---|---|
It is simple to implement and easier to interpret. | Look at only linear straight-line relationships. |
Linear regression analysis offers less complexity to establish relationships between independent and dependent variables. | Often assumes that there is a straight-line relationship between variables. |
Linear regression analysis offers less complexity to establish relationships between independent and dependent variables. | Often assumes that there is a straight-line relationship between variables. |
Improves marketing strategies and handles multiple variables at a time. | Observes the mean of a dependent variable. |
Supports concept and product testing with better prediction and forecasting. | Not useful when actual mathematical relationships between known variables. |
Applicability
- Customer retention: It allows you to measure newly acquired customers’ retention and purchasing power compared to those not enrolled in loyalty programs.
- Program impact: Determining how loyalty programs influence the buying trends and average spending of customers per purchase.
8. Monte Carlo Simulation
Monte Carlo Simulation (MCS) is a method of data analysis that employs random sampling to identify complex mathematical or statistical problems. The main objective of MCS is to quantify uncertainty and assess risks. By modelling the scenarios, MCS gives insights into potential volatility and outliers.
The MCS method assumes that input probability distributions can easily be estimated. The quality of results mainly depends on these input distributions. Furthermore, MCS is a versatile method used to find applications in finance for valuing complex portfolios, in engineering for system reliability assessments, and project management for risk analysis.
Advantages | Limitation |
---|---|
Flexible for complex systems. | Solutions are statistical by nature. |
Provides accurate results when proper implementation of analysis is done. | High precision comes at a high computational cost. |
Incorporates realistic models. | Results may have statistical errors, requiring multiple runs for reliability. |
Efficient for certain problems. |
Applicability
- Marketing campaign: Monte Carlo Simulation can help you determine a new marketing campaign based on your target audience and preferences, which will likely raise sales.
- Some other fields, like environmental studies, use this method for prediction and modelling purposes.
Read more: How to Become a Junior Data Analyst: Beginner’s Guide
9. Factor Analysis
The factor analysis method defines the variations with observed related variables that depend on lower unobserved variables termed factors. It helps in extracting independent variables that are ideal for the optimisation of specific segments.
For example, if you have any product and collect customer feedback, these types of data analysis methods will help you focus on specific factors like layouts, potential errors, product performance, and current trends. These factors can vary according to your objectives.
Advantages | Limitation |
---|---|
Reduces data complexity. | Requires a large sample size. |
Identifies hidden factors and uses them to predict future outcomes. | Results lead to overfitting of data. |
Identifies hidden factors and uses them to predict future outcomes. | Results lead to overfitting of data. |
Simplifies interpretation by grouping variables. | Does not handle missing data, outliers and categorical data well. |
Applicability
- Service improvement: Identifying key factors like wait time, staff behaviour, and treatment outcome impact patient satisfaction.
- Resource allocation: Using these insights will improve areas that would significantly affect patient satisfaction.
10. Time Series Analysis
A time series analysis technique checks data points over a specific timeframe. You can use this method to monitor data within a particular time frame on a loop. However, this method is not ideal for data collection in a specific time interval.
This technique is ideal for identifying how each variable is dependent, how the result was achieved, and how the variable changed with the interval. Furthermore, you can rely on time series analysis to determine market trends and patterns over time. You can also use this method to forecast future events based on the insights from the derived data.
Advantages | Limitations |
---|---|
It helps in forecasting data by uncovering patterns in data helpful for predicting future data points. | Requires a large historical dataset. |
It has data cleansing filters and removes unnecessary noises, outliers and provides a clean analysis. | Adjusts slowly to changes in sales. |
It helps in analysis of data and gives its true meaning via autocorrelation patterns and seasonality measures. | Because of large illustrations in current data, the forecasting process faces many errors. |
Applicability
- Demand forecasting: Helps estimate sales volume based on historical sales data.
- Resources planning: Allows for the adjustment of production schedules and inventory levels to meet anticipated demands.
Read more: Top 10 AI Tools for Data Analytics
Conclusion
Now that you have understood what are the methods of data analysis, it’s time to apply these techniques to significantly impact organisations. Data analysis methods provide the mechanism for transforming raw data into insights to build better strategies.
Though the ever-growing array of techniques seems daunting, they are easy to employ when you have clear conceptual and technical knowledge and skill sets. So, get ready and give it a shot.
Master the art of data analysis with our Data Analytics & Engineering Bootcamp! Gain hands-on experience and expert guidance to excel in this ever-evolving field.
FAQs
A: To choose the right method, first define your objectives (e.g., identifying trends, forecasting, or decision-making). Then assess your data type (structured or unstructured) and complexity. Use descriptive analysis for summaries, predictive for forecasting, and prescriptive for actionable decisions.
A: For large datasets, techniques like machine learning, cluster analysis, and big data analytics are effective. Tools like Apache Spark, Hadoop, or cloud-based platforms can efficiently handle and analyze massive data volumes.
A: Use predictive analytics when you want to forecast future trends, such as customer behavior, sales, or market conditions. It’s especially useful in industries like finance, retail, and healthcare for proactive decision-making.
A: Exploratory data analysis (EDA) uncovers patterns and insights from raw data without pre-defined hypotheses, while confirmatory data analysis (CDA) tests specific hypotheses using statistical techniques to validate assumptions.
A: Real-time data analysis processes data instantly as it’s generated, providing immediate insights. Traditional methods analyze historical data, which may lead to delayed decisions. Real-time analytics is ideal for industries like e-commerce and IoT.
A: Tools like Tableau, Power BI, Python, R, and SAS support advanced methods. For machine learning and AI-driven analytics, platforms like TensorFlow, Azure ML, and Databricks are highly effective.
A: Tools like Tableau, Power BI, Python, R, and SAS support advanced methods. For machine learning and AI-driven analytics, platforms like TensorFlow, Azure ML, and Databricks are highly effective.
A: Qualitative methods focus on non-numerical insights, which may be subjective and hard to generalize. They also require manual interpretation, which can be time-consuming and prone to biases.
A: Data cleaning ensures accuracy, consistency, and completeness of data. Without cleaning, errors like duplicates or missing values can skew results and lead to incorrect conclusions.
A: Visualization transforms raw data into charts, graphs, and dashboards, making it easier to interpret insights. It helps stakeholders quickly understand trends, patterns, and anomalies.
A: For unstructured data (e.g., text, images, or videos), methods like text mining, natural language processing (NLP), and deep learning are highly effective in extracting meaningful insights.