Business Intelligence vs Artificial Intelligence: Know the Differences [2025]
- -
- Time -
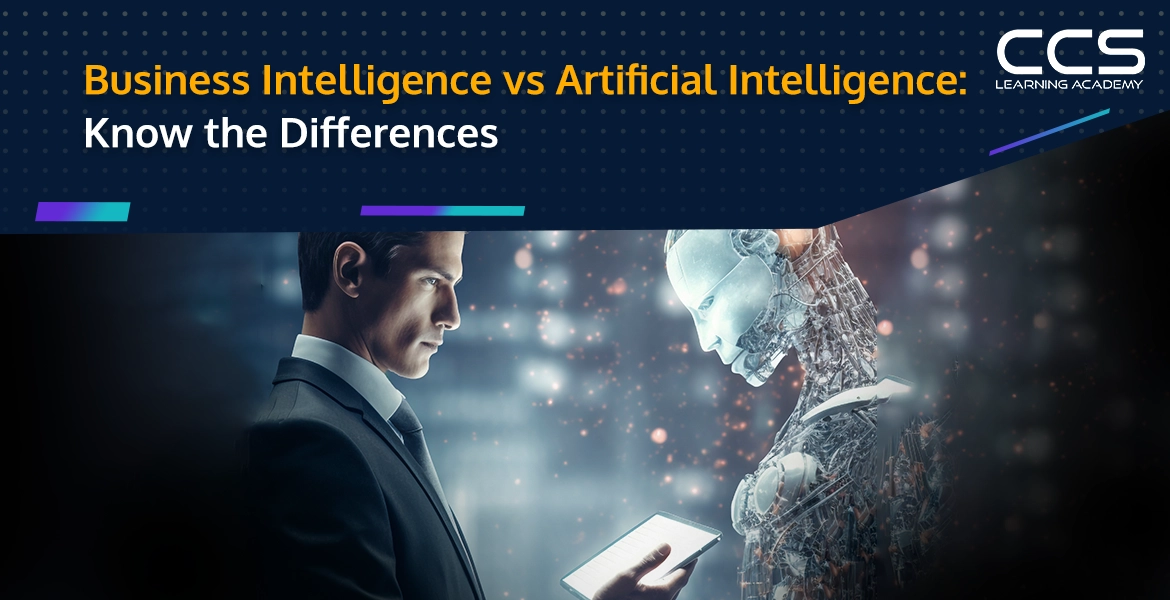
Business intelligence (BI) and artificial intelligence (AI) have become ubiquitous buzzwords in the business world. While both technologies involve analyzing data to gain insights, they have distinct objectives and approaches. Understanding the differences between BI and AI is crucial for organizations to choose the right solutions and leverage their combined power.
This article aims to provide a comprehensive overview of BI and AI – their definitions, key components, use cases, differences, and how businesses can integrate them. It also explores emerging trends in each field and offers practical guidance to determine the most suitable solutions based on specific needs and goals. By the end, readers will have a clear understanding of BI versus AI and how to strategically apply these transformative technologies.
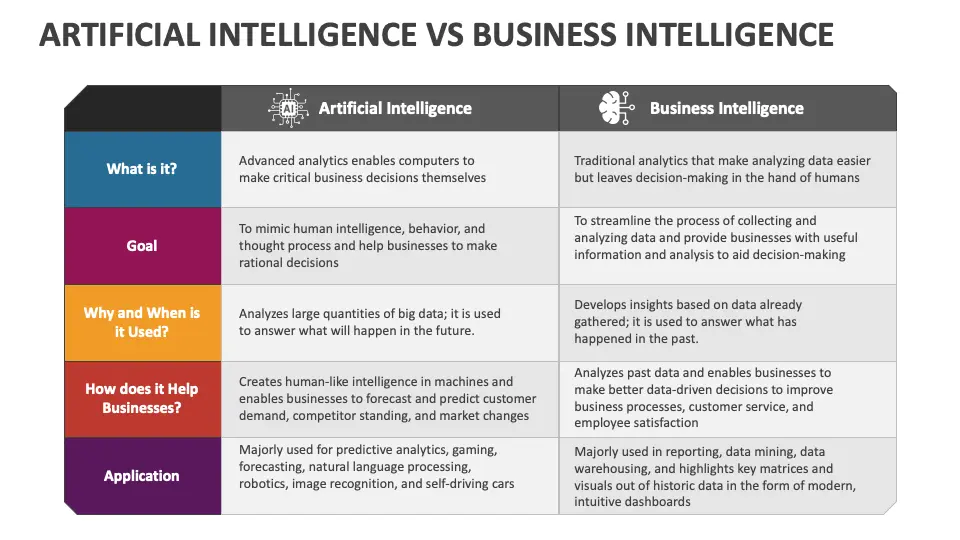
Understanding Business Intelligence (BI)
Business intelligence refers to technologies and tools used to gather, store, analyze, and provide access to data to help business users make more informed decisions. The core goal of BI is to transform raw data into actionable insights by analyzing historical trends and relationships.
The main components of typical BI systems include:
- Data warehousing – A centralized repository for integrating and storing vast amounts of historical data from various sources.
- Data integration – The process of extracting, transforming, and loading data from source systems into the data warehouse.
- Data mining – The process of analyzing large datasets to group and identify patterns using statistical and machine learning algorithms.
- Reporting – Generating reports on key performance indicators (KPIs), financials, operations, etc. based on aggregated and analyzed data.
- Dashboards – Visual tools that display the most vital/real-time metrics and KPIs via charts, gauges, and displays.
Popular BI tools across various platforms include Microsoft Power BI, Tableau, Qlik, Domo, SAS, IBM Cognos, and MicroStrategy. While open-source options like Pentaho and Grafana are also gaining traction. Each has its strengths for data modeling, visualization, and report functionalities.
Use cases of BI in various industries
BI finds extensive applications across industries like retail (inventory analysis, demand forecasting), healthcare (clinical analytics, population health management), and financial services (compliance, fraud detection, risk analysis). Overall, it helps industries optimize operations, monitor performance, and enhance decision-making through data-driven insights.
Understanding Artificial Intelligence (AI)
Artificial intelligence refers to the simulation of human intelligence processes by machines, especially computer systems. These systems are designed to think and act rationally to achieve specific goals and carry out human-like tasks.
The major techniques that enable AI include:
- Machine learning – The ability of algorithms and systems to learn from data using statistical techniques and progressively improve performance on tasks without being explicitly programmed.
- Deep learning – A branch of machine learning where neural networks inspired by the human brain are trained on vast amounts of data.
- Natural language processing – Enables machines to understand, process, and generate human languages to answer questions or summarize text.
- Robotics – Involves designing robots and algorithms to perform physical tasks autonomously in unpredictable environments.
Popular tools and platforms for developing AI applications include TensorFlow, PyTorch, Scikit-learn, Keras, SpaCy, and many others supported by cloud providers like AWS, Azure ML, and Google Cloud AI.
Use cases of AI in various industries
AI is being applied across industries like automotive (autonomous vehicles, predictive maintenance), healthcare (drug discovery, medical diagnostics), customer service (chatbots, virtual assistants), advertising (personalized recommendations, dynamic creative optimization), and manufacturing (quality control, predictive maintenance). Overall, AI automates decision-making, gains deeper customer insights, and optimizes core operations.
Key Differences Between BI and AI
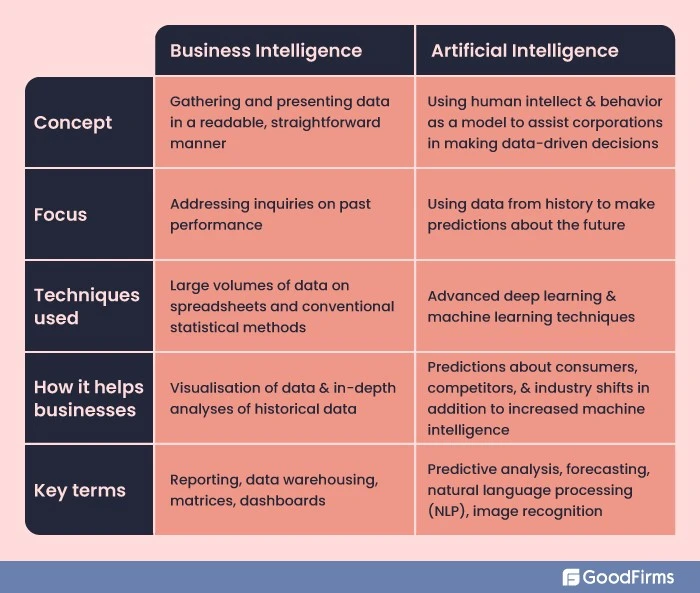
Business intelligence (BI) and artificial intelligence (AI) are two of the most transformational technologies organizations leverage today to gain valuable insights from data. While both aim to analyze data and draw conclusions to support decision-making, BI and AI differ significantly in their approach, capabilities, and outputs. Let’s delve deeper into the key variances between these complementary but distinct disciplines.
Purpose
To kick things off, it’s important to unpack the fundamental purpose each technology serves. At its core, BI primarily focuses on looking back at historical data to better understand what has previously taken place and gotten us to this point. By digging into the past through reporting and visualization of structured data stored in dimensional models, BI tools allow users to peek into prior performance and see what has transpired up until now.
AI, on the other hand, emphasizes using vast amounts of data to train sophisticated algorithms that can foresee likely future trends, outcomes, and scenarios. Through machine learning and predictive analytics, AI platforms aim to anticipate what may unfold going forward and prescribe optimized actions through autonomous decision-making.
Technology
Moving beyond purpose, the two technologies also diverge sharply in the type of technology that powers their analytics. Traditional BI solutions typically leverage only structured data that has been neatly arranged and modeled in dimensions and facts for simplified reporting and querying using SQL. This restricts analysis to what is contained in pre-configured data models.
By contrast, cutting-edge AI is adept at not only handling structured information but also unstructured data like text, audio, and images. AI leverages machine learning algorithms, neural networks, and deep learning techniques to uncover deeply hidden patterns in massive amounts of complex and unstructured data – an ability conventional BI falls short of.
Output
Naturally, these disparities in purpose and underlying technology translate to dissimilar outputs between BI and AI as well. The principal outcome of standard BI includes dashboards, scorecards, pivot tables, and formatted reports configured to provide visibility into past performance. While insightful for reviewing what has been, these retroactive visualizations only allow users to analyze historical data through a rear-view mirror.
AI, on the other hand, generates forward-looking perspectives with predictions of upcoming trends, personalized recommendations, and automated decision-making through imitation of human intelligence. Rather than exclusively living in the past, AI prescriptions aim to guide strategic decisions for the future.
Input Data
BI mainly relies on structured historical data from internal sources like transactions, sales records, financial reports, inventory levels, etc. stored in data warehouses or data lakes.
Processing Style
BI involves analytical processing where data is queried and analyzed using mostly predefined algorithms, calculations, and visualizations to generate reports.
AI leverages machine learning and deep learning algorithms that can autonomously learn from large amounts of data, recognize patterns, and then make data-driven predictions and recommendations without being explicitly programmed.
Tools
Popular BI tools include Tableau, Power BI, QlikView, IBM Cognos, and Microsoft SQL Server Reporting Services (SSRS), which provide visualization, reporting, and dashboarding capabilities.
Some common AI and machine learning development platforms include TensorFlow, Keras, PyTorch, sci-kit-learn, Apache SparkMLlib, and Microsoft Azure Machine Learning Studio. Specialized AI services from cloud majors are also popular.
Skill Requirements
BI implementation requires skills in data warehousing, ETL processes, querying languages like SQL, and visualization tools. Storytelling skills are also crucial for BI.
AI projects demand strong proficiency in algorithms, math, statistics, data science, and programming for machine learning model building and deployment. Deployment also requires DevOps skills.
Applications
Key applications of BI include financial reporting, sales & operations analysis, customer behavior analysis, risk management, compliance, and more.
AI is finding widespread use in areas such as predictive maintenance, fraud detection, forecasting, personalization, automation, computer vision, medical diagnostics, autonomous vehicles, and more.
While BI and AI seem quite different based on these parameters, there is actually a positive synergy emerging between the two domains. Many organizations are now leveraging a combination of BI and AI capabilities for enhanced decision-making.
Here are some ways they are bringing the powers of these technologies together:
- Building AI solutions on top of existing BI platforms, tools, and data infrastructure for quicker deployment.
- Leveraging AI techniques like machine learning on historical BI data for more accurate predictions and simulations of future outcomes.
- Generating actionable insights from BI reports using AI-powered natural language processing and automation.
- Developing virtual/augmented reality and conversational BI solutions using AI for immersive analytical experiences.
- Continuous monitoring of KPIs/OKRs using BI and course corrections based on AI forecasting and prescriptive analysis.
- Personalized and dynamic data storytelling using BI visuals along with AI language generation capabilities.
User Interaction
Last but not least, BI and AI diverge noticeably in how end users interact with each system. Traditional BI tools are engineered to be exceedingly user-friendly and easy for a variety of business professionals to tap into without extensive technical skills.
AI platforms, on the other hand, involve advanced data science and call for technical know-how to implement and maintain machine learning models and deep neural networks. Typically requiring data scientists and engineers to work behind the scenes, AI relies more heavily on specialized expertise than BI’s egalitarian access.
Integration of BI and AI
While Business Intelligence (BI) and Artificial Intelligence (AI) have traditionally operated as distinct fields, an emerging consensus recognizes their complementary relationship and the benefits of an integrated approach.
Where BI lays the groundwork for understanding historical trends and current performance, AI builds upon this foundation by introducing predictive capabilities, recommendations, and automated processes. By combining their respective strengths, organizations can leverage the full potential of data analytics to derive deeper insights, inform strategic decisions, and optimize operations.
Complementary relationship
BI provides an essential retrospective analysis of structured data through reporting, visualization, and descriptive statistics. Its focus on summarizing past business performance through Key Performance Indicators (KPIs) and dashboards gives leadership a snapshot of how the organization has been doing. This clarity into historical patterns and trends acts as a baseline for AI systems to learn from.
Integrating AI allows organizations to take BI a step further by using machine learning algorithms to detect patterns in datasets, both structured and unstructured. By analyzing what has occurred, AI can make educated guesses about what will transpire.
Leveraging its predictive prowess, AI infuses BI solutions with foresight. Tools enhanced with AI are able to automatically churn through past data and flag anomalies or new, interesting features for further examination. Analysts no longer have to manually scour reports and scour around for outliers. Instead, alerts surface only the most pertinent updates. This streamlines workflows and frees up valuable time for more strategic tasks.
AI-powered platforms also autonomously generate personalized, dynamic visualizations and recommendations based on probabilistic modeling of consumer behavior, seasonal fluctuations, and emerging needs.
Beyond descriptive capabilities, AI delivers predictive and prescriptive analytics. It predicts future outcomes, forecasts trends, segments customers, and optimizes processes based on learned models – tasks beyond the scope of basic BI. Combining BI data management and reporting infrastructure with AI predictive strength yields enhanced analytical outcomes.
By underpinning machine learning with an established BI foundation, inconsistencies and gaps in training data are reduced. AI systems benefit from high-quality, granular inputs to refine forecasts and recommendations. Automated workflows reduce manual labor costs while optimized decisions impact bottom lines.
Examples of integrated solutions
Leading companies have already begun embracing the union of these technologies. Salesforce Einstein leverages AI and ML algorithms to deliver enhanced customer relationship management through their CRM platform. Einstein augments sales and service workflows with an AI assistant, predictive lead scores, customized recommendations, and automated processes.
This empowers representatives and fosters stronger customer connections. Anthropic pairs AI/ML with supply chain data on their blockchain platform. By incorporating past sales, operations, and cost metrics, their solution predicts demand fluctuations and proposes cost-saving adjustments to inventory levels and transportation routes in real time.
Augmented analytics vendors such as Microsoft Power BI and Tableau are partnering with AI specialists to develop “embedded analytics.” These products fuse ML directly into data preparation and visualization processes. Drag-and-drop tools learn user analytics habits over time and offer dynamic, tailored interfaces with interactive natural language queries, auto-generated visualizations, and automated report delivery. Rather than viewing static dashboards, managers spend more time implementing AI-backed decisions to pull meaningful insights from their information overload.
Benefits of integration
In their integration of BI and AI, organizations strive for symbiotic relationships where each enhances the other. BI acts as the oil that fuels AI, providing focus and context through retrospective oversight. AI serves as the engine, transforming static data examination into active foresight through continuous learning, probing, and optimization recommendations.
Organizations that grasp how to leverage this synergy position themselves at the cutting edge of data-powered transformation and differentiation. While separate disciplines, BI and AI achieve their greatest potential through combination – a union that will increasingly define 21st-century business success.
Future Trends in BI and AI [2025]
Some notable BI trends include self-service BI increasing through no-code/low-code interfaces, real-time analytics and streaming becoming mainstream, and cloud-based/SaaS BI solutions gaining prominence.
In AI, areas like privacy-centric techniques, edge and distributed AI, boost in predictive maintenance through IoT/IIoT, AI for computer vision and robotics will dominate. Explainability, accountability, and oversight for trustworthy AI will be emphasized.
Combining emerging BI and AI trends, industries are expected to have more personalized, automated, and intelligent systems. Manufacturing will see major operational efficiency with predictive quality controls. Healthcare will have AI-driven disease screening, drug discovery will be more efficient. AI and IoT will optimize transportation, logistics, and supply chains.
Choosing the Right Solution for Your Business
- Factors to consider: When selecting BI or AI solutions, businesses must evaluate their data assets and technology maturity, goals like descriptive vs predictive needs, technical expertise availability, data security, compliance, and privacy requirements.
- Scenarios favoring BI: BI remains indispensable for businesses that need retrospective analysis of key metrics, forecasting based on historical trends, integrating diverse data sources, and providing intuitive data access for decision-makers.
- Scenarios favoring AI: Organizations seeking to automate decision-making, generate highly accurate personalized insights at scale, or optimize complex processes in real-time using pattern recognition stand to gain more from AI technologies.
- Considerations for combined adoption: Businesses looking to incorporate both need data-driven cultures and roadmaps, data engineering expertise, hybrid cloud platforms for governance and scale, upskilling teams on evolving techniques, and focus on responsible AI best practices.
Conclusion
In conclusion, while BI and AI differ in approaches, together they form a synergistic whole that leverages the extensive experience in descriptive analytics from BI and introduces the human-like decision-making, automation, and predictive powers of AI. Organizations must judiciously adopt the most suitable solutions by clearly understanding their unique needs and continuously evolving these potent technologies for competitive advantage.
The future undoubtedly belongs to enterprises that can successfully integrate BI and AI to unlock unprecedented insights, efficiencies, and opportunities through data-driven decision-making and continuous learning systems. Those who pioneer this strategic approach will lead their industries by pioneering the next wave of digital transformation.
Master both Business Intelligence (BI) and Artificial Intelligence (AI) with CCS Learning Academy’s comprehensive courses. Our BI course teaches you to analyze historical data and create insightful reports using tools like Tableau and Power BI. The AI course covers machine learning and predictive analytics with frameworks like TensorFlow and PyTorch.
Gain hands-on experience, practical training, and expert guidance to excel in these dynamic fields. Enroll now at CCS Learning Academy and lead the next wave of digital transformation.
FAQs
Business Intelligence (BI) refers to technologies, applications, strategies, and practices used to collect, integrate, analyze, and present business information. The purpose of BI is to support better business decision-making through the use of historical data and reporting tools.
Artificial Intelligence (AI) involves creating computer systems that can perform tasks that typically require human intelligence. These tasks include learning, reasoning, problem-solving, perception, and language understanding. AI is about building systems that can learn and make decisions independently.
BI is primarily about using data-driven insights to inform decision-making, focusing on descriptive analytics to create reports and dashboards that illustrate past or current trends. AI, on the other hand, uses algorithms and machine learning to perform tasks that adapt and learn from their data inputs, focusing more on predictive and prescriptive analytics.
BI tools include data visualization software, data warehousing systems, and dashboards (e.g., Tableau, Power BI). AI technologies involve machine learning frameworks, natural language processing tools, and neural networks (e.g., TensorFlow, PyTorch).
BI is used to enhance business operations through better data organization, analysis, and reporting. Common applications include performance measurement, enhancing customer engagement, budgeting, and financial forecasting.
AI applications are broad and include automating routine tasks, providing personalized customer experiences, optimizing logistics, detecting fraud, and powering innovations like autonomous vehicles and smart assistants.
BI tools are primarily used by business analysts, data analysts, and executive management who need to make informed decisions based on historical data. AI is used by a wider range of professionals including software engineers, data scientists, marketing teams, and product managers to create systems that can predict and adapt to future scenarios.
Careers in both fields are growing. BI professionals can expect roles like BI Analyst, BI Project Manager, or BI Developer. AI offers opportunities as AI Engineers, Data Scientists, Machine Learning Engineers, or AI Researchers. AI tends to command higher salaries due to its technical complexity and the advanced math skills required.
Both BI and AI play crucial roles in business strategy. BI provides the groundwork for strong data handling and analysis capabilities which are essential for any data-driven organization, while AI offers advanced capabilities that can lead to significant competitive advantages through innovation and efficiency.
Companies don’t necessarily have to choose one over the other; rather, they should assess their immediate needs and long-term goals. BI is essential for understanding past and current business performance, making it indispensable for daily operations. AI, however, may be more of a strategic investment that drives innovation, efficiency, and long-term growth.