Will AI Replace Data Engineers? Understanding the Future of Data Roles
- -
- Time -
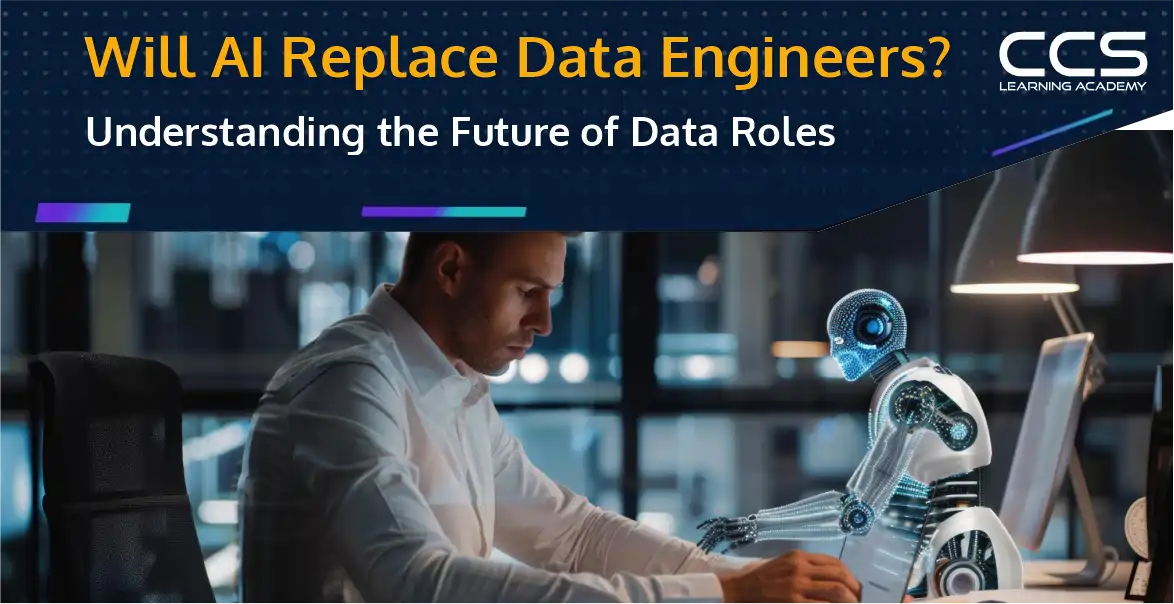
A lot of chatter has been revolving around artificial intelligence (AI) advancing to replace data-related jobs. But is it a reality or just a hype? The expertise provided by humans in data roles can be matched with effort. Yes, it is definitely true that AI has extreme potential to change the world’s tech infrastructure.
AI adds value to the landscape created by data engineers and data scientists. These data roles, like data engineers and data scientists, are needed in the workplace to oversee the process and build language models to make AI understand and work.
Data engineers are the ones who know how to consume a model and plug it into a data pipeline for the automation of value extraction. To understand the sparkling question of will AI replace data engineers, let’s have a look at this brief guide.
Table of Contents
- What is the Role of Data Engineers?
- 3. Implementation
- What is the Current Scenario of Data Roles?
- How Does AI Impact Data Engineering?
- Will Data Engineers Become Obsolete in the AI Age?
- Why Won’t AI Replace Data Engineering?
- How Do AI and Data Engineers Work Together?
- The Future Landscape of Data Engineers with AI
- FAQs
What is the Role of Data Engineers?
Data engineers gather data according to the business requirements and evaluate them to design meaningful insights, which later can be implemented in decision-making by the stakeholders and managers. Furthermore, they use data-based information to build a data pipeline, system, and framework for future reference.
The entire working of data engineers happens through the following process:
1. Planning
This is phase 1 of data engineers functioning. Here, they understand current data and gather more data based on requirements. They define key performance indicators (KPIs) to set the planning process into motion.
AI’s Role:
In planning, AI can help data engineers gather and analyze data. It may also streamline some tasks that do not need critical thinking or human insight.
2. Designing
In the designing phase, engineers choose the exemplary architecture and framework that enables the prediction of costs and improves scalability. They assess tools to identify which tool suits best for the project they are working on.
AI’s Role:
AI can help data engineers evaluate tools and predict costs while suggesting scalable solutions. It can also analyze large chunks of data to benchmark the existing tools. However, the final selection decision heavily relies on human expertise and judgment.
3. Implementation
The implementation phase includes building data pipelines and frameworks based on the project requirements. Data engineers face challenges in delegating tasks and writing exact codes that they have planned. Comprehensive documentation is also needed, which might delay the data engineering process.
AI’s Role:
AI can help manage different tasks simultaneously and delegate tasks using tools like GitHub, Copilot, etc. AI can also handle coding tasks and testing and even generate documentation for data engineers to ease their workload and productivity.
4. Operation
The operation phase is the last phase, during which system monitoring is done carefully. Identifying errors, fixing bugs, and training staff are other essential operations to improve data infrastructure.
AI’s Role:
AI is already proving its worthiness in monitoring systems and predicting potential errors before they appear to humans. Automatic bug fixing through machine learning is another solution provided by AI to enhance system reliability. However, human intervention remains crucial for strategic improvements and problem-solving.
By understanding what data engineers need to do and will AI replace data engineers, we can say that AI and humans should work side-by-side, enhancing overall efficiency and productivity.
What is the Current Scenario of Data Roles?

AI and machine learning have long been part of the tech world. But recently, in 2024, AI has gained the attention of the world. The development of AI toolboxes like ChatGPT has sparked the potential of technology. Meanwhile, data professionals are working to upskill their expertise with AI. They understand the rising demand for AI and how AI could assist them in their job to improve their efficiency.
Though AI is increasing, data professionals are also upskilling and getting ready to handle this technology effectively. Various organizations have now started focusing on their technology infrastructure and thus require data professionals to bring value to their business.
By 2030, demand for data professionals is expected to grow by an impressive 36 percent. So, no matter how AI models advance, they can’t have analyst skills like critical thinking, ethical judgment, or emotional intelligence that add value to business.
How Does AI Impact Data Engineering?
The rise of AI is reshaping world technology and thus changing the professional landscapes of data-related jobs where data engineers are directly experiencing its impact. Data engineering and AI seem like two fields, but their symbiosis can’t be denied. The role of data engineering is crucial for building essential models, and AI is built on high-quality data. Both are best in their job, and when integrated, AI can vastly enhance the data engineers’ tasks.
So, the answer to “Will AI replace data engineers?” is no, as they have much more potential to work together and transform the data engineering models. However, here is how AI is already transforming data engineering:
Automated Code Generation
Generative AI has been developed, allowing the creation of a model that can quickly generate ETL codes like SQL queries or Python scripts. These ETL codes help to perform data extraction and automation tasks. The automation effectively reduces the time and effort data engineers might have required to develop those pipelines.
Data Transformation Assistance
AI is assisting data engineers in designing data transformation tasks. It can generate code snippets or rules by simply providing descriptions or examples of desired transformation.
Improves Data Quality
AI also identifies and rectifies data quality issues while the ETL process is ongoing. For example, it generates code to clean and standardize data by reducing errors. This way, AI improves data quality without any human intervention.
Scalability
With AI and machine learning models, data engineers effectively create ETL pipelines that can quickly adapt the changes to data sources as per the requirement. This flexibility increases the data processing efficiency and enables data engineers to derive meaningful insights promptly.
Will Data Engineers Become Obsolete in the AI Age?
With the world continuously changing, the technological landscape is rapidly evolving to the forefront of innovation. The most lingering question among data professionals is, will AI replace data engineers? It is a valid concern given the evolution of AI and the increasing reliance of humans on automated machines in organizations. Today, data management and data analysis techniques have evolved to their very height with AI assistance.
However, the reality of AI and its impact on data engineers is just nuanced. AI cannot be a fear of data professionals; instead, it can act as a backbone of technological maturity. So, in the coming ages, AI might better assist data engineers in solving unique challenges and automating redundant tasks. This will save time and effort for data engineers and help them focus on more productive things.
If you want to boost your skills and learn data engineering, join the CCSLA Snowflake Data Engineer Training program or DP-203T00-A: Data Engineering on Microsoft Azure course to clear your concepts, and don’t miss any opportunity.
Why Won’t AI Replace Data Engineering?
As we understand, AI cannot be a fear but a boon to data engineers. Here are the reasons why AI won’t be able to replace data engineering or any other data roles:
1. Limited Artificial ‘Intelligence’
AI can’t think like humans. It needs more critical thinking and rationality. Writing proper code and handling different tasks at a time doesn’t showcase the ability to do everything. Certain things within organizations need a human touch.
Similarly, intelligence goes beyond just responding to queries. It requires creativity, knowledge, and emotional intelligence. No matter how much data or information you give AI, it always regurgitates what it has learned. AI cannot create abstract thoughts like data engineers on its own; it needs to be fed.
2. AI Needs to Understand Business Requirements
A data engineer must grasp every aspect of business, including its issues, challenges, and requirements. The fundamentals of data engineering can easily be fed and understood by AI, but how to design it according to business requirements is better left to data engineers.
To understand business issues and needs, data engineers converse with the organization’s individuals and pay close attention to department challenges. This helps them create genuine solutions in response to the requirements. However, AI can be used to speed up the process as AI tools support automation, preventing laborious work.
3. Lacks Comprehensive Skill
AI is now configured to generate specific results. Analyzing, automation, and deployment are some areas in which AI offers the best results. However, some contexts require human expertise, like who will use the code and how it will generate better responses. It requires understanding how the model will affect the system and data pipeline structure.
Though AI will help write codes, data engineers can create more intricate and abstract work with their critical thinking, emotional intelligence, and problem-solving skills. Data engineers create value in an organization by understanding the contexts and responses.
How Do AI and Data Engineers Work Together?
AI and data engineers can work together to create the most reliable solution for an organization.
Here are the ways in which both AI and data engineers can work:
Automation and AI Integration
The future of data engineering will see a surge in automation and integration with AI tools. Automation will help streamline repetitive tasks, which allows them to focus on more complex and strategic planning. AI algorithms will be essential in optimizing data processes and enhancing predictive analytics.
Cloud-native Solutions
With continued improvements and expansion of data volumes, the scalability of projects can be established. AI integration in data engineers’ roles will create cloud-native solutions. This will become more prevalent in the coming years, enabling organizations to improve data infrastructure. It will facilitate flexibility, cost-efficiency, and accessibility for data engineering processes.
Data Governance and Security
It is essential to address privacy concerns and data security within organizations. Future data engineering practices incorporated with AI assistance can create a strong emphasis on the data governance framework. Organizations should invest in AI tools effectively and develop strategies with data engineers to maintain data integrity, compliance, and security.
Data Storage Diversity
Since many data types exist, organizations should have secure and diversified storage solutions. Data expansion is growing, and traditional data storage methods may not be relevant. Therefore, the adoption of NoSQL databases and any other specialized storage system is needed. This diversity will help choose the best storage solution for their data types.
Ethical Considerations in Data Engineering
The future of data engineering will see a growing concern of ethical considerations as AI will help them in automation and other tasks. Data handling becomes easy with AI; the organization will focus on ethical data processing, collection, and analysis practices. Data engineers will have to follow ethical guidelines, promoting transparency and trust with stakeholders.
Continuous Integration and Deployment (CI/CD)
The future of data engineering sees wide adoption of CI/CD practices in data pipelines. CI/CD are borrowed principles from software development and are the approach that aims to streamline the development and deployment process. It ensures faster and more reliable updates. Integrating CI/CD in data engineering processes will enhance data teams’ collaboration and agility and reduce errors.
The Future Landscape of Data Engineers with AI
The lingering question of will AI replace data engineers marks fear among data engineers and other data professionals. However, understanding the above concepts, we can say that data engineers face no fear of losing their jobs but should see AI as a significant tool that can enhance their job outlook in the future.
The demand for data engineers is expected to grow as businesses heavily rely on data-driven approaches, and they need data professionals. Given the automation, AI integration, and improved scalability, the data engineering field will likely undergo revolutionary change.
Therefore, adopting this new change must begin. If you aspire to be a data professional, start your preparation and shape yourself to suit the criteria of being a data professional. You can fuel up your skills and knowledge with CCSLA’s Analytics & BI Courses. It will amplify your expertise and demand in the future, expanding the lucrative opportunities in various industries.
FAQs
AI is increasingly being used to automate routine data management tasks such as data cleaning, integration, and transformation. However, rather than replacing data engineers, AI is enhancing their productivity and allowing them to focus on more complex and strategic tasks within the data pipeline.
While AI can automate many repetitive and well-defined tasks, it is not currently capable of fully replacing the nuanced decision-making and problem-solving abilities required in data engineering. Complex tasks involving judgment, planning, and managing unexpected issues in data systems still require human oversight.
Data engineers should focus on enhancing skills that AI cannot easily replicate, such as critical thinking, strategy development, and complex problem-solving. Skills in AI and machine learning itself are also valuable, enabling engineers to work alongside AI tools and leverage them effectively.
The role of data engineers may evolve to include more emphasis on designing and maintaining AI-driven automation systems. This could shift their focus from manual data manipulation to overseeing the data architecture required to support sophisticated AI models and ensuring these systems deliver accurate and reliable outputs.
Integrating AI into data engineering can lead to more efficient data workflows, reduced errors in data processing, and faster time-to-insight for decision-making. AI can handle large volumes of data at a speed that far exceeds human capability, allowing organizations to scale their data operations effectively.
Potential challenges include the need for continuous training to keep skills up-to-date, ensuring the quality and accuracy of AI-processed data, and managing issues of transparency and accountability in automated systems. Additionally, the integration of AI can lead to significant changes in team dynamics and workflow processes.
Ethical considerations include concerns about job displacement for routine roles, biases in AI algorithms affecting decision-making processes, and the transparency of AI-driven decisions. Ensuring ethical use of AI involves rigorous testing, validation of AI outputs, and maintaining a human-in-the-loop system where critical decisions are concerned.
Companies can prepare their teams by investing in training and development programs focused on advanced AI and data science skills. Encouraging cross-disciplinary skills and understanding of AI applications in data engineering can also empower engineers to adapt to evolving roles.
The future for data engineers looks promising despite AI advancements, as demand for skilled professionals to oversee, refine, and innovate AI data systems continues to grow. Data engineers who adapt to these changes by acquiring new skills and learning to leverage AI will likely find their roles more strategic and impactful.
Data engineers should proactively seek out opportunities to work with AI technologies and participate in relevant training and professional development activities. Staying informed about industry trends and maintaining a flexible approach to new tools and methodologies will be crucial for thriving in an AI-enhanced job market.